Introduction:
Embark on a journey exploring the digital realm, unraveling the intricacies of the Deep Fake Algorithm. This exploration aims to demystify AI-Generated Videos, shedding light on innovation, challenges, and ethical considerations. Join as we unveil mechanisms powering synthetic content, providing a comprehensive understanding of deep fake technology’s landscape.
List of Deepfake Algorithms:
Generative Adversarial Networks (GANs):
Generative Adversarial Networks (GANs) are a revolutionary concept in artificial intelligence, conceived by Ian Goodfellow and his team. These networks consist of two components, a generator, and a discriminator, engaged in a continuous duel. The generator crafts synthetic data, while the discriminator discerns between real and generated data. This adversarial collaboration propels the creation of highly realistic content, making GANs a cornerstone in the development of deepfake technology.
Autoencoders:
Autoencoders, a staple in unsupervised learning, encode input data into a condensed representation and decode it back. This neural network architecture, including variants like Variational Autoencoders (VAEs), is integral to deepfake technology. Autoencoders play a pivotal role in understanding and mimicking complex data patterns, contributing significantly to the synthesis of realistic and diverse content.
Deep Neural Networks (DNNs):
Deep Neural Networks (DNNs), particularly Convolutional Neural Networks (CNNs) and Recurrent Neural Networks (RNNs), form the backbone of deepfake algorithms. These networks analyze and synthesize facial features, expressions, and movements with a depth that enables the creation of visually convincing AI-generated videos.
Face Swap Algorithms:
Face Swap Algorithms, a specialized category within deepfake technology, focus on seamlessly swapping faces between individuals in videos. By identifying and manipulating facial landmarks, these algorithms enable the intricate task of replacing one person’s face with another, contributing to the deceptive capabilities of deepfake videos.
DeepDream:
DeepDream, a creation of Google, may not be exclusively designed for deepfakes but has found a place in altering and enhancing images. Operating on principles of convolutional neural networks, DeepDream has been adapted for artistic modifications, offering a unique perspective on image and video manipulation.
Neural Style Transfer:
Neural Style Transfer algorithms, inspired by the work of Gatys et al., enable the transfer of artistic styles from one image to another. While not designed explicitly for deepfakes, these algorithms have been applied creatively to modify the appearance of faces in videos, adding artistic or deceptive elements.
Summary:
Generative Adversarial Networks (GANs) spearhead deepfake technology, engaging in a continuous battle between generation and discrimination for realistic content. Autoencoders, with their encoding and decoding prowess, contribute to the diversity of AI-generated data. Deep Neural Networks (DNNs), including CNNs and RNNs, form the core architecture, analyzing and synthesizing facial intricacies. Face Swap Algorithms focus on seamless facial exchanges, adding to the deceptive capabilities of deepfakes. While DeepDream offers artistic alterations, Neural Style Transfer creatively transforms images with different styles. Together, these algorithms define the landscape of deepfake creation, blending creativity, ethics, and technological sophistication.
Decoding the Deep Fake Algorithm: Unveiling the Technology Behind AI-Generated Videos
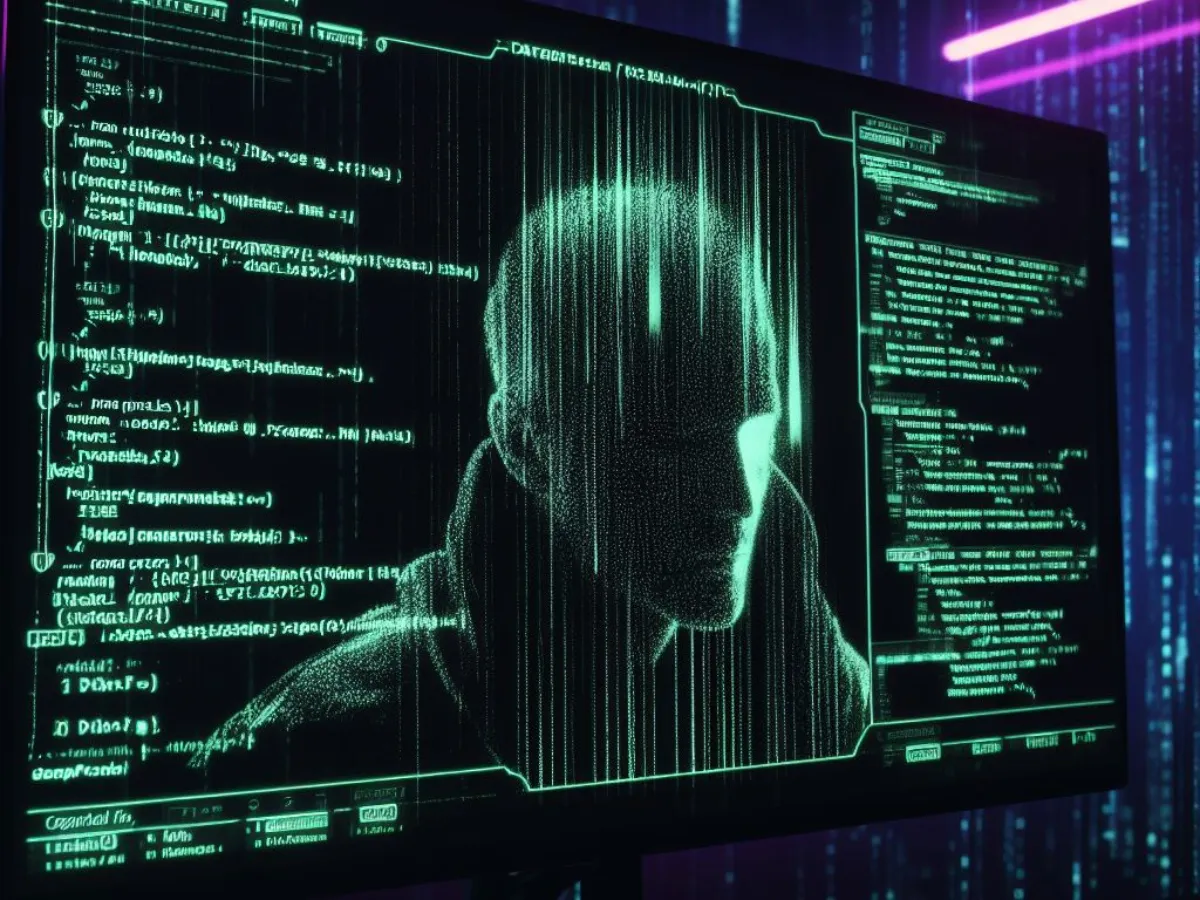
In the realm of AI-generated videos, understanding the genesis of deep fake technology is crucial. Delving into its historical perspective unveils a journey that began with the convergence of artificial intelligence and media manipulation. The roots trace back to the early 2010s, as researchers explored the potential of deep learning in creating realistic visual content.
The Genesis of Deep Fake Technology: A Historical Perspective
The inception of deep fake technology marked a paradigm shift in digital content creation. Researchers initially focused on refining facial recognition algorithms, paving the way for the manipulation of facial expressions and gestures. As the technology progressed, deep learning models evolved, incorporating neural networks capable of generating lifelike videos by synthesizing facial features and expressions seamlessly.
The transformative journey continued, with notable milestones such as the introduction of Generative Adversarial Networks (GANs). GANs, a breakthrough in AI, enabled the creation of more convincing deep fakes by pitting two neural networks against each other—one generating synthetic content, and the other discerning its authenticity. This adversarial process elevated the sophistication of AI-generated videos, leading to the realistic portrayal of individuals in entirely fabricated scenarios.
As deep fake technology matured, it transcended the realm of academia, finding applications in various industries. From entertainment to political discourse, the impact of AI-generated videos became increasingly pervasive, prompting a closer examination of the underlying architecture that brought them to life.
Behind the Code: Understanding the Architecture of Deep Fake Algorithms
To comprehend the intricacies of AI-generated videos, a close inspection of the underlying code is imperative. Deep fake algorithms, at their core, leverage neural networks with multiple layers. These networks, often convolutional neural networks (CNNs) and recurrent neural networks (RNNs), process vast datasets to learn and mimic human-like facial movements and expressions.
The architecture involves an encoding-decoding process, where the algorithm encodes facial features from a source image and decodes them onto a target face. This intricate dance of data manipulation allows the algorithm to seamlessly graft the source facial expressions onto the target individual, resulting in a video that appears authentic to the unsuspecting eye.
Ethical considerations arise as this technology becomes more sophisticated. As we unveil the layers of code behind deep fake algorithms, it’s crucial to navigate the moral landscape and explore the ethical dilemmas associated with the creation and dissemination of AI-generated content.
Ethical Dilemmas in AI-Generated Content: Navigating the Moral Landscape
As we decode the deep fake algorithm, ethical considerations loom large. The ability to manipulate videos with such precision raises questions about the responsible use of AI-generated content. One primary ethical dilemma revolves around the potential misuse of deep fake technology for malicious purposes, such as spreading misinformation, fabricating evidence, or damaging an individual’s reputation.
Navigating this moral landscape requires a careful examination of the intentions behind creating deep fakes. While the technology offers creative possibilities in entertainment and digital art, its misuse poses significant threats to societal trust and security. Striking a balance between innovation and responsibility becomes paramount in ensuring the ethical development and deployment of AI-generated videos.
Detecting and addressing the ethical concerns associated with deep fake technology necessitate a robust understanding of the strategies for identifying these undetectable manipulations. With the increasing sophistication of AI, strategies for deep fake algorithm identification become essential tools in maintaining trust and integrity in digital media.
Detecting the Undetectable: Strategies for Deep Fake Algorithm Identification
As we venture further into the exploration of deep fake technology, the need to detect these undetectable manipulations becomes a critical aspect of responsible digital engagement. Various strategies and technologies have emerged to identify AI-generated videos, providing a countermeasure against the potential harms associated with their misuse.
Forensic analysis, a cornerstone in the identification process, involves scrutinizing the video for inconsistencies in facial expressions, lighting, and shadows. Deep fake detection tools utilize machine learning algorithms trained on vast datasets of both real and manipulated videos, enabling them to discern subtle differences that escape the human eye.
The integration of blockchain technology also emerges as a potential solution, offering a transparent and tamper-proof ledger of video authenticity. Collaborative efforts between technology developers, researchers, and policymakers play a pivotal role in establishing standardized protocols for deep fake identification, ensuring a collective defense against the deceptive capabilities of AI-generated content.
While the quest for detection strategies progresses, a fundamental question arises: How do we differentiate between real and synthetic content, and what impact does this distinction have on the way society perceives information through media?
Real vs. Synthetic: Analyzing the Impact of AI-Generated Videos on Media Perception
In the landscape of digital media, the advent of AI-generated videos challenges our traditional understanding of reality. Analyzing the impact of these synthetic creations on media perception requires a nuanced examination of how they shape public discourse, influence opinions, and contribute to the evolving landscape of information consumption.
AI-generated videos introduce a layer of complexity to the media ecosystem, blurring the lines between truth and fiction. The seamless integration of fabricated content into our visual experience raises concerns about the potential erosion of trust in the authenticity of digital media. As viewers encounter increasingly convincing deep fakes, the ability to discern reality becomes a valuable skill in navigating the digital landscape.
Media literacy, therefore, becomes a cornerstone in mitigating the potential negative impact of AI-generated videos on public perception. Empowering individuals to critically evaluate the content they encounter is essential in fostering a society that can distinguish between real and synthetic narratives.
The artistry and scientific precision behind facial reenactment, a key technique in deep fake technology, add another layer of intrigue to the discussion. A closer look at the nuances of facial reenactment reveals the amalgamation of artistic expression and technological sophistication that defines this aspect of AI-generated videos.
The Art and Science of Facial Reenactment: A Close Look at Deep Fake Techniques
Facial reenactment stands as a testament to the seamless integration of art and science within the realm of deep fake technology. This technique involves the meticulous recreation of facial expressions and movements, blending the artistic intent of the creator with the scientific precision of the underlying algorithms.
At its core, facial reenactment relies on the algorithm’s ability to analyze and understand the intricacies of facial features. By mapping key points on a source face and transplanting them onto a target face, the algorithm achieves a level of realism that challenges traditional notions of visual authenticity.
The artistic aspect of facial reenactment emerges in the careful curation of expressions and emotions. Creators can manipulate not only the visual appearance of a person but also the emotional undertones conveyed in the AI-generated video. This blending of artistic intent with technological capability introduces a new dimension to storytelling and digital expression.
AI-generated videos extend their influence beyond mere technological marvels; they play a pivotal role in revolutionizing the film and gaming industry, offering unprecedented possibilities in character creation, storytelling, and immersive experiences.
AI-Generated Videos in Entertainment: Revolutionizing the Film and Gaming Industry
The impact of AI-generated videos extends far beyond ethical considerations and technological intrigue; it is reshaping the landscape of entertainment. In the film industry, deep fake technology opens avenues for casting decisions, allowing filmmakers to recreate iconic characters or bring historical figures to life with unprecedented realism.
The gaming industry, known for its pursuit of cutting-edge technology, embraces AI-generated videos to enhance character animations and storytelling. Deep fake algorithms enable the creation of lifelike characters that respond dynamically to player actions, providing a level of immersion previously thought unattainable.
While the entertainment industry reaps the creative benefits, the proliferation of deep fake technology also introduces security risks and potential harms to individuals and organizations. Safeguarding the digital landscape requires a comprehensive approach to mitigating risks posed by deep fake algorithms.
Securing the Digital Landscape: Mitigating Risks Posed by Deep Fake Algorithms
As we unravel the intricacies of deep fake technology, the conversation inevitably turns to the risks associated with its widespread use. Safeguarding the digital landscape requires proactive measures to mitigate the potential harms posed by malicious applications of AI-generated videos.
One primary concern lies in the potential for deep fake technology to be weaponized for disinformation campaigns, political manipulation, or cyber threats. By creating convincing yet fabricated content, bad actors can exploit vulnerabilities in information systems, leading to real-world consequences.
Mitigating these risks involves a multi-faceted approach, combining advancements in technology, policy frameworks, and public awareness. Collaboration between tech developers, cybersecurity experts, and policymakers is essential in developing robust defenses against the malicious use of deep fake algorithms.
While the risks are real, understanding how AI-generated videos impact social media and online interaction is equally important. Exploring user perspectives sheds light on the ways in which individuals navigate the digital landscape in the era of deep fake technology.
User Perspectives: How AI-Generated Videos Impact Social Media and Online Interaction
The influence of AI-generated videos extends to social media, shaping the way individuals interact and consume information online. User perspectives play a pivotal role in understanding the nuanced dynamics of social interactions within the digital realm, especially as the prevalence of deep fake technology continues to rise.
On one hand, AI-generated videos offer new avenues for creative expression and digital storytelling. Users engage with entertaining and innovative content that blurs the lines between reality and fantasy. However, on the other hand, the potential misuse of deep fake technology introduces challenges related to trust, authenticity, and the reliability of information circulating on social media platforms.
Navigating this landscape requires a combination of user education, platform policies, and technological interventions. Platforms must implement measures to detect and mitigate the impact of AI-generated content on user experiences while fostering an environment that encourages responsible digital citizenship.
As we examine the user perspectives, it becomes evident that the continual evolution of deep fake algorithms poses both challenges and opportunities for the future. Exploring the innovations and potential pitfalls in the ongoing development of this technology unveils the frontiers that lie ahead.
Future Frontiers: Innovations and Challenges in the Continual Evolution of Deep Fake Algorithms
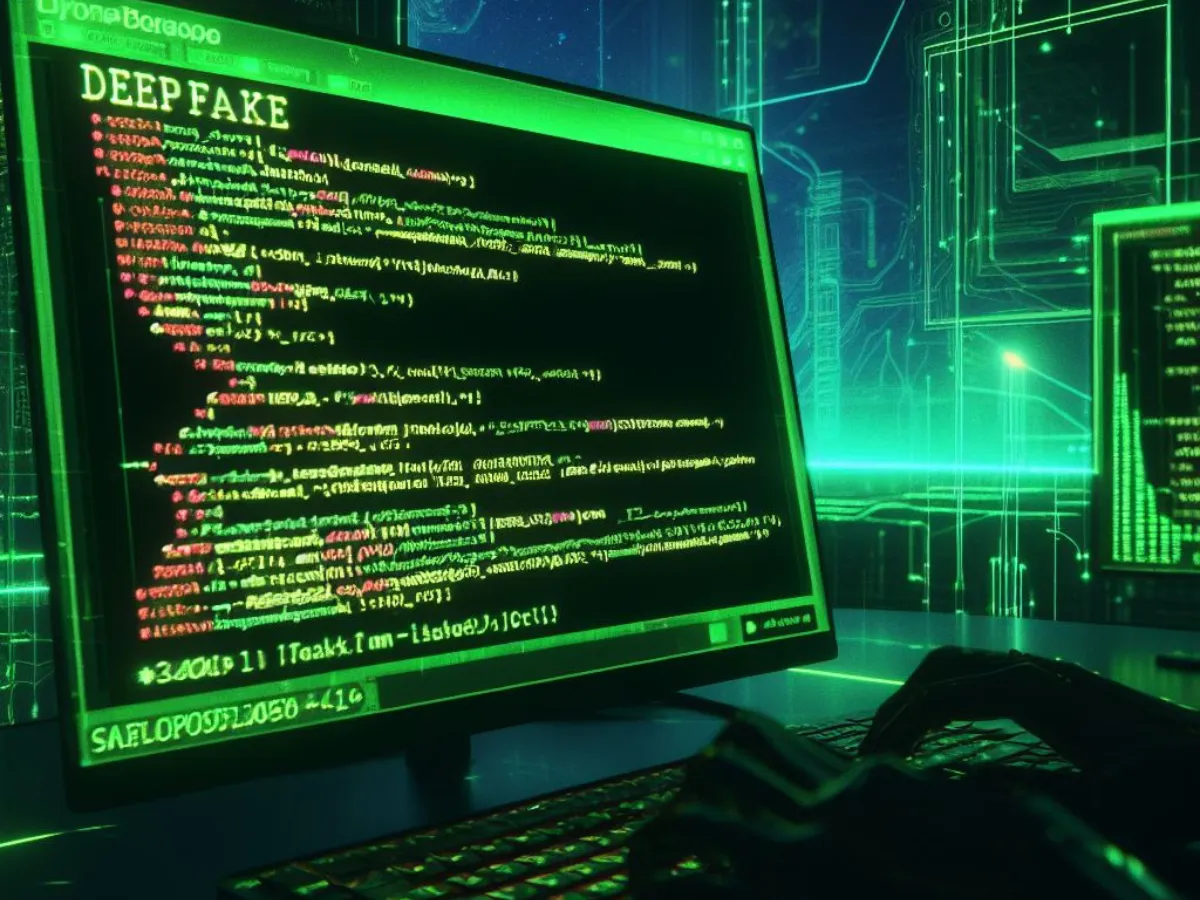
In the continual evolution of deep fake algorithms, future frontiers beckon with both promise and challenges. Innovations in artificial intelligence promise even more realistic and sophisticated AI-generated videos, blurring the lines between the authentic and the synthetic.
Advancements in machine learning, coupled with increased computational power, may lead to the democratization of deep fake technology. As accessibility grows, so do the challenges of mitigating potential risks and ensuring responsible use across diverse sectors.
Striking a balance between fostering innovation and safeguarding against malicious intent becomes paramount. Ethical considerations must guide the development and deployment of deep fake algorithms, with a focus on preserving trust, transparency, and the integrity of digital media.
Conclusion
In the intricate landscape of AI-generated videos, our journey of decoding the deep fake algorithm unveils a captivating intersection of technology, ethics, and societal impact. From its historical roots to the present-day sophistication, deep fake technology represents a powerful tool that demands both awe and scrutiny. As we delve into the architectural intricacies, the fusion of art and science in facial reenactment, and the transformative influence on entertainment and social media, a nuanced understanding emerges.
Ethical considerations echo loudly, urging responsible innovation to safeguard against potential misuse. Strategies for detecting these undetectable manipulations become vital, emphasizing the need for collaborative efforts to uphold the integrity of digital content. Exploring user perspectives underscores the dynamic interaction between individuals and AI-generated content, highlighting both the creative potential and the challenges of navigating a digitally manipulated landscape.
In contemplating future frontiers, the balance between innovation and ethical safeguards becomes paramount. As the continual evolution of deep fake algorithms unfolds, a call to action resonates — to navigate this technological terrain with a conscientious mindset, fostering responsible use, preserving trust, and embracing the transformative potential while guarding against its potential pitfalls. The unveiling of the deep fake algorithm’s technology demands a collective commitment to shaping a digital landscape that balances innovation with ethical responsibility.